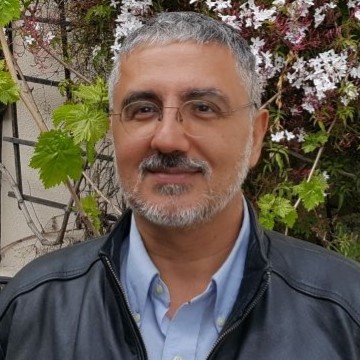
Computational Hypergraph Discovery, a Framework for Connecting the Dots
Houman Owhadi
Approved for Public Release
We propose the development of a comprehensive, interpretable computational framework de-signed to radically simplify and transform the resolution of most complex problems within and be-yond the scientific domain. This ambition mirrors the transformative impact of calculators on arith-metic, with the potential to reshape the boundaries of what we perceive as achievable in Science. Unlike prevalent empirically driven research, it will come with simple and transparent theoreti-cal and computational guarantees. While these objectives may appear overly ambitious, they can be achieved by developing a framework for completing and discovering of computational hyper-graphs. These hypergraphs are generalized graphs representing functional dependencies between groups of variables (node clusters) and functions through directed hyperedges linking these clus-ters. They offer a natural platform for organizing, communicating, and processing computational knowledge. While most Computational Sciences and Engineering (CSE) and Scientific Machine Learning (SciML) problems can be framed as the data-driven discovery of unknown functions in a computational hypergraph whose structure is known, many problems in Science require the data-driven discovery of the structure (nodes and connectivity) of the hypergraph itself. Here, we plan to master the completion and discovery of computational hypergraphs through a generaliza-tion of Gaussian Process (GP) and, more broadly, of (possibly non-Gaussian) Optimal Recovery (OR) methods inheriting the guarantees of kernel/OR methods. Hypergraph discovery is enabled by the UQ capabilities of GPs (and of OR methods) used as a sensing mechanism for overcom-ing the curse of combinatorial complexity. Our approach contrasts sharply with the complexity of causal inference methods for such problems, which grows superexponentially with the number of variables. By enabling polynomial complexity in hypergraph discovery, this sensing mechanism, absent in ANN-based methods, unlocks immediate application at an unprecedented scale across various domains, including reasoning with raw data (identifying a network of hidden relationships between variables without a parametrized model), intelligence gathering and material/chemical re-action discovery. We aim to evolve this framework towards applications of extreme complexity emerging from both concrete and abstract applications encompassing high-dimensional nonlinear singular black-box systems, algorithm discovery, the co-design of computing platforms, and the discovery of new forms of computation.