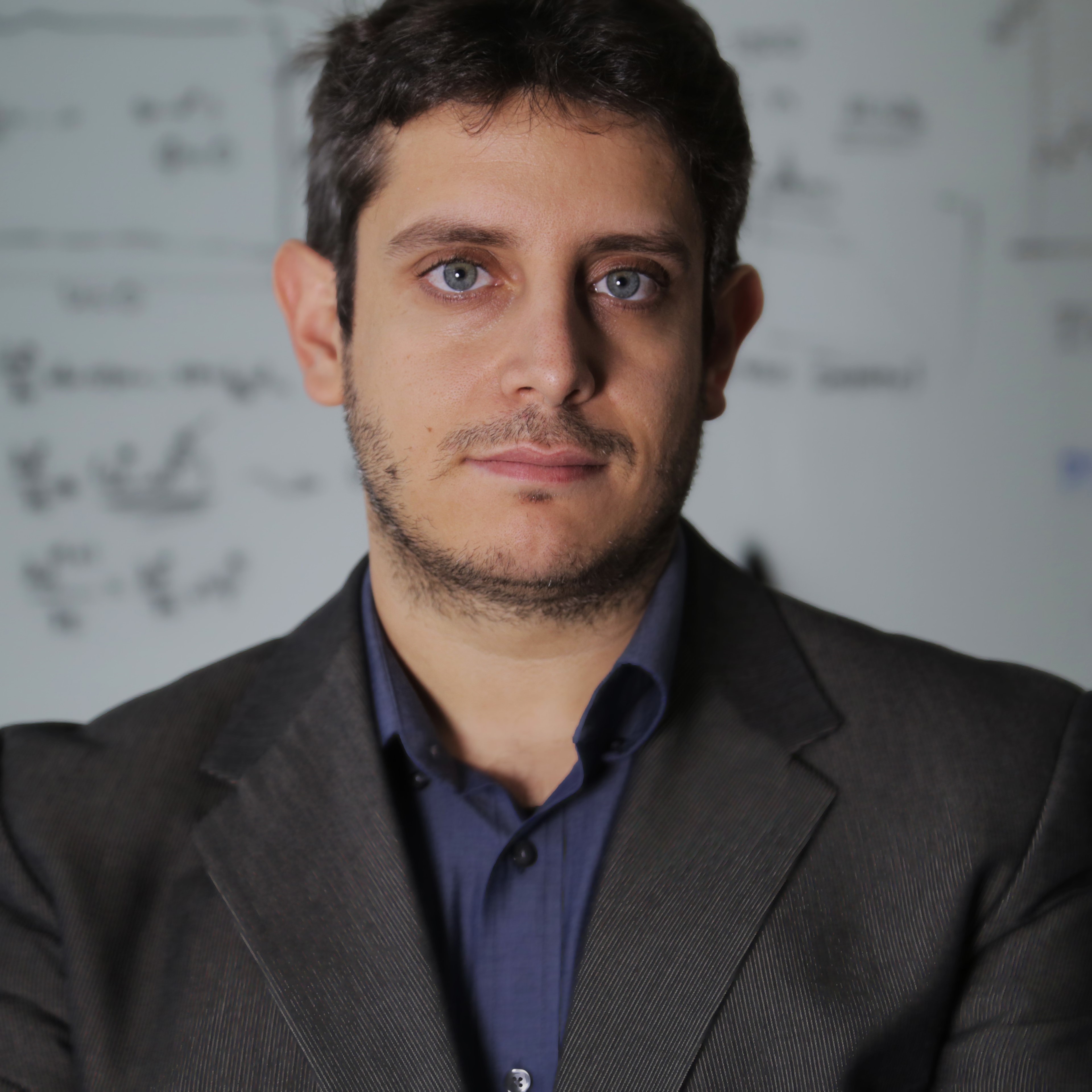
Generative Active Modeling Along Unstable Dynamics for Capturing Unprecedented Extreme Events
Themistoklis Sapsis
Approved For Public Release
This project aims to advance fundamental knowledge on the algorithms and theory for the efficient computation of extreme event probabilities, precursors and design of mitigation strategies in complex dynamical systems. For a plethora of systems relevant to the DoD we have stochastic dynamics characterized by strongly transient features that rise without obvious warning. These transient extremes, although rare, can be catastrophic and therefore it is essential to forecast them, mitigate them if possible, and quantify the probability of their occurrence in the long term. Typical examples include risk quantification for extreme events in human-made systems (e.g. aeroelastic instabilities), extreme climate events such heat waves and cascade effects to interconnected systems, but also mission-critical algorithmic problems such as search and path planning operations for extreme anomalies.
We plan to formulate and apply ideas rooted in generative algorithms in the context of extreme events by constraining the generation of scenarios consistently with the instabilities of the dynamical system. The motivation for this direction is the aim to generate new, potentially unseen, extreme events, which will provide unprecedented capabilities for prediction, statistical modeling and quantification. The extreme event generators will be complemented with an extreme-event-aware learning method that will result in not only dynamics-consistent scenarios of extreme events but also probabilistically feasible, i.e. non-exotic. The new generative learning approach will be combined with a new class of data- and model-selection criteria for extreme events that will dynamically optimize the training criteria, so that errors associated with rare events are automatically detected and penalized accordingly.
The proposed framework is motivated by real-world problems in fluids and mechanics, modeled by continuous and discrete systems, on which we plan to validate and assess our methods. We expect that the proposed effort will provide educational opportunities for students and postdocs who can later be part of the defense workforce, but also lead to new areas for future research aligned with the DOD’s current and projected future challenges.