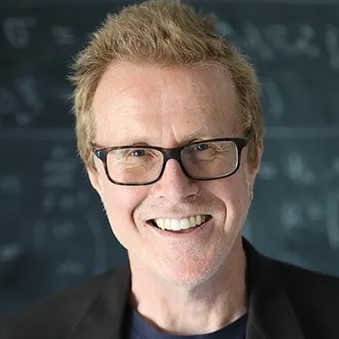
Andrew Stuart, California Institute of Technology
Abstract
(Approved for public release)
The proposed work addresses the urgent need for the development of a mathematical framework for the analysis and development of model-centric and data-informed predictive computational science and engineering; this will allow for the integration of centuries of mathematical modeling experience, decades of computational modeling experience, and recent innovations in algorithms, computer design and hardware stemming from the current revolution in the data sciences. Creating this framework requires a new theory at the interfaces of machine learning, numerical analysis and statistics. This theory will underpin computational science and engineering in the twenty first century. The proposed research will address this problem in the specific setting of the learning of mappings between spaces of functions – input-output maps. This setting has numerous applications in the solution of system-level tasks arising in science and engineering. The proposed fundamental research will be developed in tandem with applications, some of direct of interest to DoD, such as climate modeling, materials science and robotics.
The goal of the proposed research is to develop the mathematical framework needed for algorithms that accelerate execution of black box computer code, by means of a data-informed surrogate, or algorithms that discover a structured computational model when a first principles model is not available, as often arises for cyber-physical systems. The technical approach is to view the black box or cyber-physical system as an input-output map and approximate it by a cheaper (in terms of computational cost) or safer surrogate. It is natural to exploit ideas from machine learning to identify a surrogate. However, standard machine learning tools do not scale to the high dimensional input and outputs encountered in physical science applications, nor do they readily incorporate domain-specific knowledge. The novelty underpinning the approach proposed here is to view the map as acting between Banach spaces of functions, the setting needed to allow for a principled development and analysis of data-driven scalable surrogates. It is also a natural setting in which to incorporate (physical) laws representing domain-specific conceptual understanding. The approach requires development of a new theory at the interfaces of machine learning, numerical analysis and statistics. The objectives are to introduce novel designs for surrogate maps, to prove error bounds on the surrogates in order to certify computations and evaluate the relative efficiency of different methods, and to develop new data-generation processes in order to learn the surrogate maps in an optimal fashion from limited data; additional applied objectives focus on applications to inverse problems and data assimilation.
The proposed perspective on surrogate maps between spaces of functions constitutes a paradigm shift with wide-ranging anticipated outcomes over a decadal time-horizon. The work will lead to a new mathematical framework for algorithm development and analysis. And the deep understanding arising from this framework will enable, through acceleration of the repeated evaluation of the core input-output map, solution of system level tasks in key areas such as optimal design, uncertainty quantification, inversion and time-stepping. The understanding will also lead to solution of system-level tasks arising in cyber-physical systems, currently not even contemplated, through discovery of data-driven models which respect physical and mathematical principles.