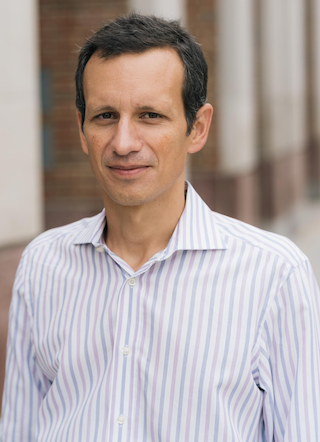
Abstract
(Approved for Public Release)
Modern science is undergoing what may arguably be called a data revolution, whereby an exponential rate of increase of computing and data acquisition capabilities, coupled with advances in machine learning, has enabled the creation of data-driven models for complex physical processes that match or exceed the skill of first-principles models. Realizing the full spectrum of benefits of this new paradigm hinges upon the development of physical and mathematical frameworks that can intrinsically handle probabilistic descriptions of complex systems in the presence of highly nonlinear dynamics. Quantum mechanics, together with its mathematical underpinnings in operator algebras and gauge theory, provides such a framework which has historically shown tremendous success in describing atomic- and subatomic-scale phenomena, but whose applicability and potential benefits in the context of classical complex systems, such as turbulent fluid flows, remain largely unexplored. In response, we propose a comprehensive research program to devise novel quantum mechanical formulations of key problems in modeling of classical dynamical systems, and create data-driven implementations of these techniques through machine learning approaches. Specifically, the proposed research will focus on (i) Learning dynamical laws from data through spectral analysis of Koopman operators on non-commutative algebras of observables; (ii) Analysis of observables with spatial structure and dynamical symmetries using gauge theory; (iii) Quantum mechanical approaches for stochastic subgrid-scale modeling and control; and (iv) Development of compilation strategies for simulation of classical dynamics on quantum computers.
Collectively, these techniques will create a new paradigm for statistical modeling of classical systems based on state-of-art approaches from fundamental physics and mathematics, realized through machine learning. A core element of this paradigm is that formulating finite-dimensional approximation schemes in non-abelian operator spaces enables preservation of intrinsic algebraic structures of classical dynamical systems, such as the Leibniz rule for vector fields, in ways which are not possible through conventional discretizations. Moreover, for systems with spatial structure, the proposed gauge-covariant methodologies will enable seamless fusion of sensor data acquired from different frames of reference, providing representations of physical configurations and laws in their intrinsic geometrical form. Looking ahead to the quantum computing era, the project will lay out the foundations for scalable, consistent quantum simulation of classical systems through encodings of Koopman operators in quantum circuits.
The proposed research program also has a strong applied component, addressing challenging current problems in climate dynamics and fluid dynamics. Areas of focus include uncertainty quantified forecasting of the El Nino Southern Oscillation, subgrid-scale modeling of convective ˜ processes, and spatiotemporal interpolation of high-resolution, along-track satellite altimetry for oceanic turbulence.
The mathematical and computational methodologies developed by the project will find wide applicability in many DoD-relevant contexts including artificial intelligence, simulation of natural and engineered systems, and quantum information. In addition, results from domain-scientific applications will impact DoD strategic and operational capabilities through improved analysis and forecasting of environmental data. The project will contribute to workforce development through interdisciplinary training of postdoctoral researchers and PhD students