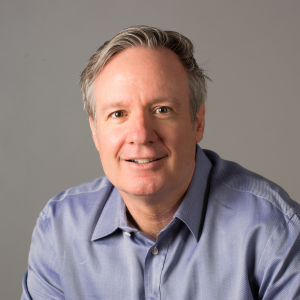
Paul Sajda, Columbia University
Approved for Public Release
ABSTRACT
In understanding how our brains generate perception, cognition and emotion, important questions are “what are the dynamics of these processes in ecologically realistic settings?” and “how might we manipulate the dynamics of these processes so as to potentially augment or optimize behavior?” In this project we address these questions by making measurements of neural activity, physiology and human behavior “in-the-wild”, and testing interventions that manipulate these dynamics for real-world tasks. Specifically, we propose a framework that engages macroscale brain circuitry central to rapid decision-making, specifically a circuit that includes the anterior cingulate (ACC), dorsal lateral prefrontal cortex (dlPFC) and locus coeruleus (LC). Our overarching scientific hypothesis is that unique functional dynamics of the ACC-dlPFC-LC, particularly those reflecting modulation by stress, are observable by linking neuro-physio-behavioral measurements from controlled laboratory settings with those conducted in naturalistic settings.
This project proposes a new approach that uses neuroimaging and neurostimulation technologies, already approved for human use, and state-of-the-art machine learning to fuse and integrate the resulting information revealed across different measurement modalities and task environments. Specifically we will develop a testable model of human brain dynamics that governs rapid decision-making in natural environments, and use machine learning, particularly deep learning, to make linkages between EEG and fMRI neuroimaging measurements and tasks that are carried out in both highly controlled laboratory environments and those that are acquired in less well-controlled, but more ecologically realistic virtual (VR) and augmented reality (AR).
Our project will develop a new computational framework that enables fusion and “transcoding” of neural and physiological measurements between different modalities and noise conditions, enabling development of a macro-scale neural circuit model that is based on high resolution neuroimaging data as well as real-world tasks and behavior. Also novel and innovative, is that we will use closed-loop, endogenously triggered, neuromodulation to test causal interactions in this circuit and how these relate to behavior. The three primary objectives of the project are,
Objective 1: Develop an experimental paradigm and instrumentation for measuring and modulating activity of ACC-dlPFC-LC circuit in both the laboratory and naturalistic environments:
Objective 2: Develop a hypothesis driven theoretical model of ACC-dlPFC-LC function based on switching multivariate dynamical systems.
Objectives 3: Develop and evaluate a computational framework for relating lab and in-thewild ACC-dlPFC-LC circuit activity, with a key innovation being development of a deep learning framework enabling “neural transcoding” - i.e. generating one neuroimaging modality from another.
In addition to shedding new light on how the human brain rapidly makes decisions in real-world situations, we believe this project will have broad implications to cognitive neuroscience as well having revolutionary impact on DoD capabilities in the areas of human-to-human and human-machine interaction. The translation of models of brain dynamics from the laboratory to real-world setting opens the door for multi-human neural integration. These models create a natural “language” by which neural systems can communicate. This communication could be both human-to-human and human-to-machine. For example, these models would facilitate human-agent teaming, by providing machine-based agents/actors with neurally-informed models of group and/or individual’s “state-of-mind” when making decisions.