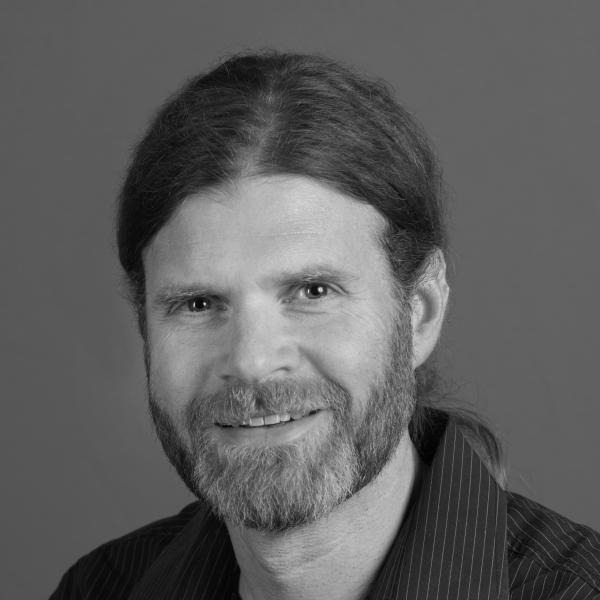
PI: David J. Freedman
The University of Chicago
(Approved for Public Release)
Abstract
The past decade has seen remarkable advances in the parallel fields of neuroscience and artificial intelligence (AI), and the pace of progress is expected to accelerate in the coming years. On the AI side, the latest generations of artificial neural networks (ANNs) have been remarkably successful in solving tasks that had previously been considered intractable. New methods for training ANNs with multiple connected layers (termed "deep" networks) have allowed these networks to autonomously drive vehicles, classify natural images, and accurately translate languages. On the neuroscience side, rapidly evolving techniques for monitoring and manipulating neural circuit activity, along with increasingly sophisticated theoretical frameworks, have led to a more mechanistic understanding of how activity in neuronal circuits gives rise to complex behaviors.
The neuroscience and AI fields have recently become increasingly intertwined, with advances in one field inspiring progress in the other. For example, until recently ANNs only bore a slight resemblance to their biological counterparts. As principles learned from studying biological networks have been incorporated into ANNs, major improvements in ANN performance have resulted. The consensus is that future progress in ANN design, and for AI more generally, will critically depend on increasingly close interplay with experimental neuroscience.
The goal of this project is to employ a combination of neuroscience experimental approaches and AI approaches for understanding the circuit computations underlying cognitive functions such as learning, memory, and decision making. The experimental approach will be to perform large-scale electrophysiological recordings and functional microscopic imaging from dense ensembles of cortical neurons in awake subjects trained to perform visually-based tasks requiring decision making, learning, and flexible switching between contexts or rules. Parallel AI work will use machine learning approaches to train recurrent ANNs on the same behavioral tasks used in the experiments, and to implement neural circuit mechanisms discovered in the neuroscience experiments in order to construct novel AI systems with enhanced cognitive capabilities. The insights gained through ANN work will also inform the approach to design of novel neuroscience experiments, generate new hypotheses for experiments, and approaches for analyzing experimental data. will This approach will yield a basic understanding of the neural network computations which allow both biological and artificial neural networks to perform complex cognitively demanding tasks.