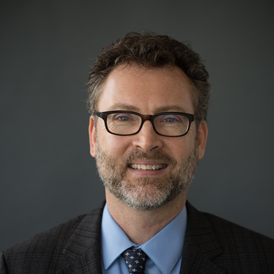
Michael D. Graham, UW-Madison
Project Summary/Abstract
(Approved for public release)
In a fluid such as air or water, turbulence arises in almost all flows in which the nearest surface or interfaces is more than about 1 millimeter away. Thus the vast majority of fluid motion in nature and technology is turbulent. Turbulent flows contain a complex combination of structure and randomness that makes understanding and predicting them one of the great challenges of the physical sciences. Two distinct approaches to such phenomena are the fields of nonlinear dynamics, which concerns the relation between observed time-dependence and the state space of a system, and machine learning, which focuses on the automated detection of meaningful patterns from data. The overall goal of the present proposal is to extend the dynamical systems approach to turbulent flow and to integrate it with ideas from machine learning to have impact on the understanding and control of complex turbulent flows. The primary focus will be plane wall-bounded channel incompressible Newtonian flow, which is chosen because many of the most important and intriguing aspects of turbulence in flows near surfaces such as airplane wings are found in this simple geometry. The work is organized into three closely intertwined aims.
Aim 1: Multiscale coherent states. The discovery of exact coherent states (ECS), nonturbulent solutions to the governing equations of fluid flow that capture key aspects of turbulent structure and dynamics, has substantially advanced our understanding of turbulence, especially near solid surfaces where turbulence is most coherent and intense. In this Aim, we will compute new ECS and test new hypotheses regarding the relationships between coherent states and turbulence. This work will elucidate the nature and structure of ECS as flow rate increases. To facilitate this Aim, we will develop and apply advanced computational approaches. The recent discovery by the PI’s group of an ECS that captures the hairpin vortex structure widely observed in turbulent shear flows forms a starting point for this aim.
Aim 2: Machine learning turbulent structure and dynamics. The machine learning paradigm of cluster analysis will generate new data-driven modal decompositions and dynamic models for turbulence. This approach enables nonlinear modal decompositions that partition state space into subdomains, enabling discrete approximations to the Perron-Frobenius and Koopman operators of the turbulent flow. Interrogation of these operators, which capture the overall dynamics of the flow, will yield new insights into mode and scale interactions in turbulent flows. We will develop methods to integrate information about exact coherent states within this framework to enrich its description of turbulent dynamics.
Aim 3: Shaping the state space of turbulence. We will build on understanding developed in Aims 1 and 2 to develop a systematic framework for understanding the nonlinear effects of open loop manipulations such as wall deformations for purposes of drag reduction. Key questions that we will address include: How does the state-space boundary between initial conditions that becomes laminar and turbulence change with excitation, and does this excitation lead to substantial reductions in turbulence itself? Taken together, these goals comprise an analysis of the global nonlinear receptivity to disturbances of unforced and forced wall-bounded flows.
Turbulent fluid motion and the related phenomena of mass and heat transport and energy consumption arise in a vast range of phenomena of DoD interest, ranging from atmospheric and oceanic phenomena to energy losses in aircraft flight to design of artificial organs. In the broadest sense, the aim of this proposal is to examine the ways in which nonlinear dynamics and machine learning, individually and in combination, will bring us new insights into this important phenomenon. Approved for Public Release