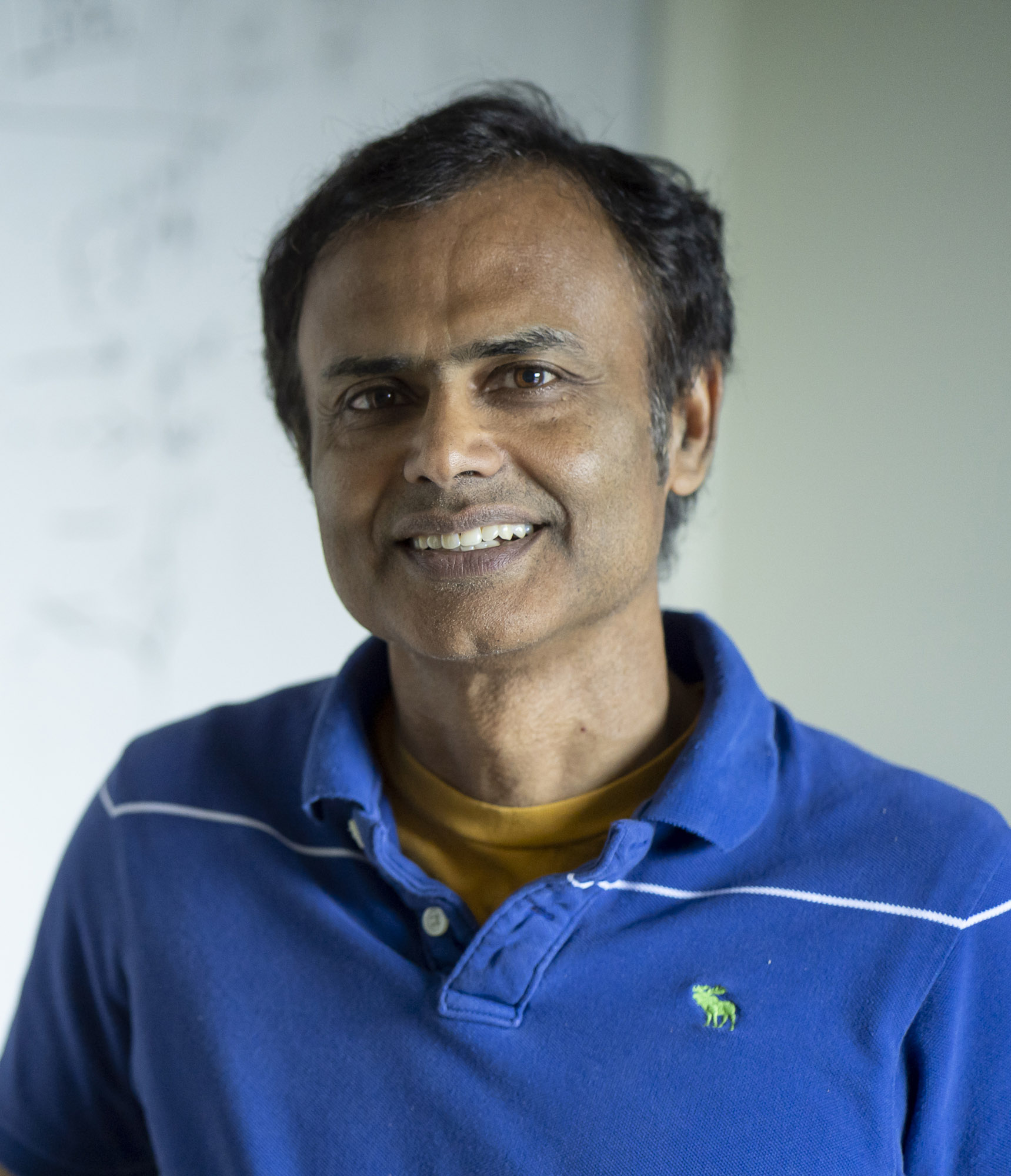
SUMMARY
The key insight behind the proposed research is that emerging devices that use spin as the physical state variable for storing information make it possible to realize neuromorphic/bio-inspired computing in a highly energy efficient manner, well beyond the capabilities of CMOS alone. This is due to two key factors – the inherent match between the characteristics of these devices and some biological functions (leading to a drastic decrease in circuit complexity), and the possibility of operation at ultra low voltages (tens of mV).
We propose a research program to investigate spin-based neuromorphic computing from the device level to new computing model/architecture. At the device level, we will develop magneto-metallic spin devices that mimic certain biological component functionality in a highly energy efficient manner. The proposed devices are based on spin-torque experiments that have demonstrated the feasibility of structures with perpendicular magnetic anisotropy, domain wall motion enhanced by spin hall effect, lateral spin valves. We will also explore systems that integrate such spin-devices with CMOS and Silicon memristors to realize complete neural network/ other biological system functionality. A devicecircuit-architecture co-simulation-framework (benchmarked against available experiments) will be developed to evaluate functionality and performance at various levels of abstraction. Our preliminary evaluations indicates the possibility of more than 100X improvements in energy consumption for applications such as image recognition, compared to state-of-the-art CMOS. The goals of the proposed research are to (i) propose devices and establish the benefits of spin based devices in the context of neuromorphic/biological computing (for which CMOS implementations are energy-inefficient), (ii) synergistically explore spin devices, circuits and architecture to augment capabilities of CMOS, and (iii) incorporate the findings in education and outreach programs.
If successful, spin devices enable new computing models at ultra-low energy consumption, mobile devices to run richer, more complex applications such as image/target recognition, video processing.